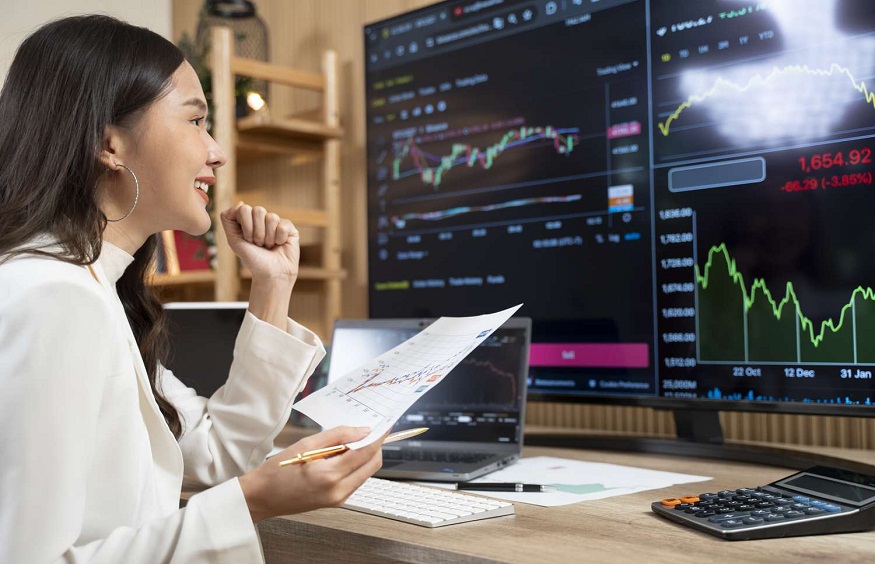
Statistical Modeling for Stock Trading: Predictive Analytics and Forecasting Methods
Stock trading has evolved significantly with the advent of data-driven technologies, and today, traders and investors rely heavily on predictive analytics and statistical models to forecast market movements. The volatility of financial markets, the complexity of trading strategies, and the speed at which data is generated demand advanced analytical tools. In this article, we explore the importance of statistical modelling in stock trading, examining key concepts, popular forecasting methods, machine learning techniques, and future trends in predictive analytics.
Understanding Predictive Analytics in Stock Trading
Predictive analytics has become a cornerstone in stock trading, offering a systematic way to forecast market behaviour based on historical data. It uses statistical algorithms and machine learning techniques to identify patterns, relationships, and trends that can help predict future price movements. In the world of stock trading, where every decision counts, predictive analytics aims to minimise uncertainty by providing more accurate and reliable forecasts.
The role of predictive analytics extends beyond simply guessing where prices will go next. It offers a scientific approach to decision-making, helping traders to assess risks, identify opportunities, and make informed choices in real-time. Predictive models in stock trading are powered by the belief that past behaviour can reveal meaningful insights into future performance, though these models require constant refinement to account for new data and changing market conditions. View more for further information.
Key Concepts in Statistical Modeling for Stock Trading
Statistical modelling in stock trading encompasses a range of techniques, all designed to help forecast stock prices, assess volatility, and improve trading strategies. Central to this concept is the idea that markets are influenced by patterns, trends, and correlations that can be discovered through data analysis. Statistical models serve as powerful tools to make sense of vast amounts of market data and convert it into actionable insights.
One of the most common applications of statistical models in trading is time series forecasting, which uses past price movements to predict future prices. This method assumes that trends and cycles will continue in some form, allowing traders to forecast short-term and long-term movements. However, predicting stock prices with absolute certainty remains impossible, as markets are influenced by a multitude of unpredictable factors. Still, statistical models allow for better-informed predictions, thus reducing risk and improving profitability.
Popular Forecasting Methods
Various forecasting methods are used in stock trading, each with its strengths and weaknesses. One of the most widely recognized methods is ARIMA, which stands for AutoRegressive Integrated Moving Average. ARIMA models are particularly effective for time series forecasting because they take into account both historical data and the residuals, or the difference between the predicted and actual values. By capturing these residuals, ARIMA models can identify and account for patterns that may not be immediately visible in the raw data. This makes them especially useful for predicting stock prices in markets with well-defined trends.
Another method commonly used by traders is the GARCH model, which stands for Generalised Autoregressive Conditional Heteroskedasticity. Unlike ARIMA, which primarily focuses on predicting prices, GARCH models are more focused on forecasting market volatility. By modelling variance and measuring the degree of uncertainty in asset prices, GARCH models help investors assess risk and make better decisions about portfolio diversification and hedging strategies. GARCH models are particularly helpful in managing the inherent volatility that characterises the stock market, enabling traders to adjust their strategies based on expected fluctuations.
Machine Learning and AI Techniques in Stock Trading
While traditional statistical models remain highly valuable in stock trading, machine learning and artificial intelligence (AI) have brought a new level of sophistication to the field. Machine learning algorithms, which are designed to learn from data and improve over time, have revolutionised stock trading by offering more flexible and adaptive methods of analysis.
Supervised learning, one of the primary techniques in machine learning, uses labelled historical data to train models. For example, a supervised learning algorithm might be fed past stock prices along with known market conditions and used to predict future movements. This method is especially powerful when large datasets are available, as it allows the model to recognize patterns and make predictions based on a variety of input variables.
Model Evaluation and Performance Metrics
To assess the effectiveness of statistical models in stock trading, it’s essential to use various performance metrics. Traditional metrics such as Mean Absolute Error (MAE) and Root Mean Squared Error (RMSE) are commonly used to evaluate the accuracy of predictive models. MAE measures the average magnitude of errors in a set of predictions, while RMSE gives more weight to larger errors by squaring them. These metrics are valuable for determining how close a model’s predictions are to actual market outcomes.
Another critical aspect of model evaluation is backtesting, which involves testing a model’s predictions against historical data to determine how well it would have performed in real-world conditions. Backtesting allows traders to refine their models, ensuring that they remain effective in a variety of market conditions. However, backtesting has its limitations, particularly when it comes to accounting for future events and market shifts that are not present in historical data.
Conclusion
Statistical modelling has become an indispensable tool in stock trading, offering traders a means to make more informed, data-driven decisions. By leveraging predictive analytics, statistical models, and machine learning techniques, traders can improve their ability to forecast market movements, assess risk, and optimise their trading strategies. However, challenges remain, including the volatility of markets, data quality issues, and the interpretability of complex models.